A new theoretical framework explores how neurons can flexibly filter out information based on how relevant it is for behavior and how costly it is to retain it.
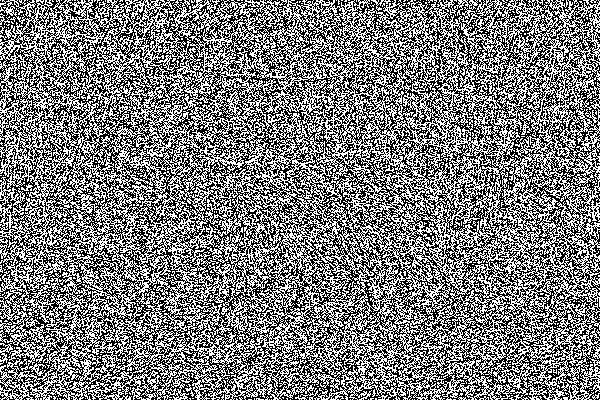
Imagine you are hiking alone in a forest, and you hear a rustle through the trees. You squint your eyes to see if you can spot something hidden in the brush, and you start to make out details of what looks like fur. Within seconds, you know there is a bear behind the trees. What do you do?
To answer this question, your brain must extract many different types of information from your sensory surroundings. Initially, you might rely on very fine visual details that can help discriminate between branches and patterns of fur. But once you know that you are looking at a bear, you likely no longer care about the details of its fur – you want to know where the bear is, and more importantly, where it’s going next.
Although you could continue tracking the details of its fur as you try to get away, this would be a waste of your energy and resources. To most efficiently use your resources, you should track only those sensory signals relevant for helping you escape, which can change over time as you gather new information from the environment.
Many animals must overcome similar challenges to survive. Their brains must make inferences about the environment by extracting information pertaining to the task at hand – be it gathering food or escaping a predator – and filtering out irrelevant information. But extracting and encoding information requires energy, and these energetic demands can increase as the behavioral task becomes more complex.
These two ideas – that animals must make inferences from incoming sensory signals, and that encoding sensory signals is energetically costly – have traditionally been explored within two separate theoretical frameworks. Because of this, it has been difficult to study how neurons make efficient use of their limited energy to infer changing properties of the environment.
In a paper published July 10, 2018, in the journal eLife, Wiktor Młynarski of the Massachusetts Institute of Technology and Ann Hermundstad of HHMI’s Janelia Research Campus bring these two ideas together within a single theoretical framework. This new framework accounts for the dynamic interplay between the energetic cost of encoding information and the relevance of that information for behavior.
A key idea is that neurons have limited resources and thus cannot retain all information from the sensory environment; some information must be filtered out. But any information that is filtered out is lost forever and cannot be used to make inferences that guide behavior. Because of this, neurons must be “smart” in choosing what information is filtered out and at which times. Młynarski and Hermundstad’s framework provides a principled account of how neurons could best solve this problem to support different behavioral goals while simultaneously dealing with uncertainty about the changing environment.
This framework makes new, testable predictions at the levels of both neuron dynamics and behavior. It shows how seemingly disparate experimental observations – for example, bursts of neural activity versus sustained responses – can be understood as different manifestations of a common set of principles.
Ann Hermundstad is a group leader at Janelia.
###
Wiktor Młynarski and Ann M. Hermundstad, “Adaptive coding for dynamic sensory inference.” eLife. Published online July 10, 2018. doi: 10.7554/eLife.32055
Bear image:
D. Martin, C. Fowlkes, D. Tal, and J. Malik, “A Database of Human Segmented Natural Images and its Application to Evaluating Segmentation Algorithms and Measuring Ecological Statistics.” Proc. 8th Int'l Conf. Computer Vision 2 (2001): 416.
*Image Credit: Ann Hermundstad/Berkeley Image Segmentation Dataset/Photo by Matstorb, CC BY 3.0